Dissertations and capstones are the culmination of years of hard work and research for students pursuing higher education. These academic ventures require meticulous planning, thorough study, and a deep understanding of the chosen subject matter. However, one crucial aspect of dissertations that often poses a significant challenge is data analysis.
Data analysis forms the backbone of any research study or dissertation. It involves the systematic examination and interpretation of data to extract meaningful insights and draw valid conclusions. Aspiring researchers must grapple with a wide array of data analysis techniques, statistical tools, and software to navigate this complex terrain successfully.
https://www.capstone-dissertationwritingservices.com/Mba-paper-506.html
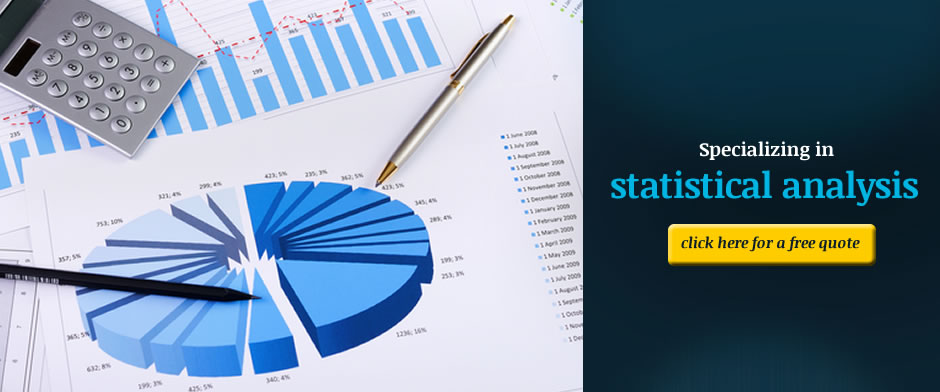
Mastering dissertation data analysis is crucial for several reasons. Firstly, it enables researchers to uncover patterns, relationships, and trends within the gathered data, providing a solid foundation for drawing accurate conclusions. Secondly, effective data analysis ensures the reliability and validity of research findings, making them more credible in the academic community. Lastly, it contributes to expanding the existing knowledge within a field of study, potentially leading to groundbreaking discoveries and advancements.
In this article, we will delve into the intricacies of dissertation data analysis, exploring key methodologies, tools, and best practices that will help researchers crack the code. Whether you are embarking on your first dissertation or looking to enhance your data analysis skills, join us as we uncover the secrets to mastering this essential aspect of academic research.
Understanding Dissertation Data Analysis
Data analysis forms a crucial component of dissertations and capstones. In order to effectively interpret and present research findings, it is essential to master the process of dissertation data analysis. This involves examining the collected data, identifying patterns or trends, and drawing meaningful conclusions. By understanding the key concepts and methods involved, researchers can ensure that their data analysis is rigorous and enhances the overall quality of their academic work.
The first step in dissertation data analysis is organizing and cleaning the data. This entails carefully reviewing the collected data to ensure its accuracy and completeness. Researchers may need to make adjustments, such as removing outliers or addressing missing values, to ensure the reliability of their results. Once the data is cleaned and organized, it can be further explored and analyzed using various statistical techniques.
Next, researchers must select the appropriate analytical methods based on their research questions and the nature of their data. This may involve employing techniques such as descriptive statistics to summarize the data, inferential statistics to test hypotheses, or qualitative analysis to interpret textual or narrative data. It is crucial to use methods that align with the research objectives and provide meaningful insights. Additionally, researchers must be aware of any assumptions or limitations associated with the chosen analytical methods.
Lastly, effective data analysis requires the ability to draw accurate and valid conclusions from the results. Researchers should critically evaluate their findings in light of the research questions and relevant literature. This includes interpreting statistical outputs, identifying significant patterns or relationships, and discussing the implications of the results. By presenting well-grounded interpretations, researchers contribute to the advancement of knowledge in their field.
In conclusion, dissertation data analysis is a vital aspect of academic research. By understanding the fundamentals of data organization, selecting appropriate analytical methods, and drawing meaningful conclusions, researchers can yield valuable insights from their data. It is essential to approach data analysis with rigor and ensure its alignment with research goals, contributing to the overall quality and significance of dissertations and capstones.
Key Methods and Techniques
When it comes to dissertation data analysis, there are several key methods and techniques that researchers use to make sense of their findings. These methods enable scholars to delve deep into the data and extract meaningful insights. In this section, we will discuss three important approaches commonly employed in dissertation data analysis.
Descriptive Analysis:
Descriptive analysis is a fundamental technique in dissertation data analysis. It involves organizing and summarizing data to gain a better understanding of its characteristics. Researchers often use descriptive statistics such as mean, median, and standard deviation to present an overview of their data. This approach allows them to identify patterns, trends, and distributions, providing a clear and concise summary of their research findings.Inferential Analysis:
Inferential analysis is another crucial method used in dissertation data analysis. This technique allows researchers to draw conclusions about a population based on a sample of data. By applying statistical tests and models, such as t-tests or regression analysis, researchers can make inferences and generalize their findings to a larger population. Inferential analysis helps researchers explore relationships, test hypotheses, and uncover significant findings in their data.Qualitative Analysis:
While quantitative analysis focuses on numerical data, qualitative analysis involves examining non-numerical data such as interviews, surveys, or open-ended responses. This method helps researchers gain a deeper understanding of the thoughts, beliefs, and experiences of their study participants. Through techniques like thematic analysis or content analysis, researchers uncover themes, codes, and patterns within the qualitative data. Qualitative analysis provides rich, contextual information that complements quantitative analysis and offers insights into the complexities of research topics.
In conclusion, mastering dissertation data analysis requires proficiency in key methods and techniques. Descriptive analysis, inferential analysis, and qualitative analysis are just a few examples of the strategies employed by researchers to analyze their data effectively. Employing these methods enables researchers to make informed interpretations, draw meaningful conclusions, and contribute to knowledge in their respective fields.
Best Practices for Dissertation Data Analysis
In order to master dissertation data analysis, there are several best practices that can guide researchers towards meaningful and insightful outcomes.
Clear Research Objectives:
Before diving into data analysis, it is crucial to have well-defined research objectives. Clearly articulate the research questions and hypotheses, as this will ensure that the analysis remains focused and aligned with the intended goals of the study.Robust Data Collection:
The quality and reliability of the data collected play a pivotal role in accurate analysis. Employ rigorous data collection methods, ensuring that the data is relevant, representative, and collected in a systematic and consistent manner. This will enhance the credibility and validity of the findings.Method Selection and Application:
Choosing appropriate analysis methods is paramount to extract meaningful insights from the data. Familiarize yourself with different statistical techniques and methodologies relevant to your research field. Then, apply these methods effectively to analyze the data, carefully considering any assumptions or limitations associated with each technique.Thorough Data Cleaning:
Raw data often contains errors, missing values, or inconsistencies. Therefore, it is crucial to thoroughly clean the data before analysis. This involves addressing missing data, identifying outliers, and resolving any inconsistencies or inaccuracies. By ensuring data integrity, researchers can avoid misleading or biased results.Organized Data Storage:
Maintain a systematic and organized approach to storing and managing data. Create a logical structure for data storage that facilitates easy retrieval and ensures data security. By establishing proper data management protocols, researchers can reduce the risk of data loss or corruption and streamline the analysis process.